Web Scraping Exercises
Tag Archives: Python web-scraping-exercises. How to Scrape all PDF files in a Website? Prerequisites: Implementing Web Scraping in Python with BeautifulSoup Web Scraping is a method of extracting data from the website and use that data for other Read More. Python BeautifulSoup. Python bs4-Exercises. This book introduces concepts and skills that can help you tackle real-world data analysis challenges. It covers concepts from probability, statistical inference, linear regression and machine learning and helps you develop skills such as R programming, data wrangling with dplyr, data visualization with ggplot2, file organization with UNIX/Linux shell, version control with GitHub,. This book introduces concepts and skills that can help you tackle real-world data analysis challenges. It covers concepts from probability, statistical inference, linear regression and machine learning and helps you develop skills such as R programming, data wrangling with dplyr, data visualization with ggplot2, file organization with UNIX/Linux shell, version control with GitHub,.
- Web Scraping Exercises Pdf
- Web Scraping Software
- Web Scraping Exercises Pdf
- Web Scraping Service
- Web Scraping Applications
- Web Scraping Software Comparison
- Web Scraping Exercises Free
Bonus materials, exercises, and example projects for our Python tutorials. Mount Olympus web scraping example app HTML MIT 3 5 0 0 Updated Dec 18, 2020.
This tutorial covers how to extract and process text data from web pages or other documents for later analysis. The automated download of HTML pages is called Crawling. The extraction of the textual data and/or metadata (for example, article date, headlines, author names, article text) from the HTML source code (or the DOM document object model of the website) is called Scraping. For these tasks, we use the package “rvest”.
- Download a single web page and extract its content
- Extract links from a overview page
- Extract all articles to corresponding links from step 2
Create a new R script (File -> New File -> R Script) named “Tutorial_1.R”. In this script you will enter and execute all commands. If you want to run the complete script in RStudio, you can use Ctrl-A to select the complete source code and execute with Ctrl-Return. If you want to execute only one line, you can simply press Ctrl-Return on the respective line. If you want to execute a block of several lines, select the block and press Ctrl-Return.
Tip: Copy individual sections of the source code directly into the console (2) and run it step by step. Get familiar with the function calls included in the Help function.
First, make sure your working directory is the data directory we provided for the exercises.
Modern websites often do not contain the full content displayed in the browser in their corresponding source files which are served by the webserver. Instead, the browser loads additional content dynamically via javascript code contained in the original source file. To be able to scrape such content, we rely on a headless browser “phantomJS” which renders a site for a given URL for us, before we start the actual scraping, i.e. the extraction of certain identifiable elements from the rendered site.
If not done yet, please install the webdriver
package for R and install the phantomJS headless browser. This needs to be done only once.
Now we can start an instance of PhantomJS and create a new browser session that awaits to load URLs to render the corresponding websites.
In a first exercise, we will download a single web page from “The Guardian” and extract text together with relevant metadata such as the article date. Let’s define the URL of the article of interest and load the rvest package, which provides very useful functions for web crawling and scraping.
A convenient method to download and parse a webpage provides the function read_html
which accepts a URL as a parameter. The function downloads the page and interprets the html source code as an HTML / XML object.
3.1 Dynamic web pages
To make sure that we get the dynamically rendered HTML content of the website, we pass the original source code dowloaded from the URL to our PhantomJS session first, and the use the rendered source.
NOTICE: In case the website does not fetch or alter the to-be-scraped content dynamically, you can omit the PhantomJS webdriver and just download the the static HTML source code to retrieve the information from there. In this case, replace the following block of code with a simple call of html_document <- read_html(url)
where the read_html()
function downloads the unrendered page source code directly.
3.2 Scrape information from XHTML
HTML / XML objects are a structured representation of HTML / XML source code, which allows to extract single elements (headlines e.g. <h1>
, paragraphs <p>
, links <a>
, …), their attributes (e.g. <a href='http://...'>
) or text wrapped in between elements (e.g. <p>my text...</p>
). Elements can be extracted in XML objects with XPATH-expressions.
XPATH (see https://en.wikipedia.org/wiki/XPath) is a query language to select elements in XML-tree structures. We use it to select the headline element from the HTML page. The following xpath expression queries for first-order-headline elements h1
, anywhere in the tree //
which fulfill a certain condition [...]
, namely that the class
attribute of the h1
element must contain the value content__headline
.
The next expression uses R pipe operator %>%, which takes the input from the left side of the expression and passes it on to the function ion the right side as its first argument. The result of this function is either passed onto the next function, again via %>% or it is assigned to the variable, if it is the last operation in the pipe chain. Our pipe takes the html_document
object, passes it to the html_node function, which extracts the first node fitting the given xpath expression. The resulting node object is passed to the html_text
function which extracts the text wrapped in the h1
-element.
Let’s see, what the title_text
contains:
Now we modify the xpath expressions, to extract the article info, the paragraphs of the body text and the article date. Note that there are multiple paragraphs in the article. To extract not only the first, but all paragraphs we utilize the html_nodes
function and glue the resulting single text vectors of each paragraph together with the paste0
function.
The variables title_text
, intro_text
, body_text
and date_object
now contain the raw data for any subsequent text processing.
Usually, we do not want download a single document, but a series of documents. In our second exercise, we want to download all Guardian articles tagged with “Angela Merkel”. Instead of a tag page, we could also be interested in downloading results of a site-search engine or any other link collection. The task is always two-fold: First, we download and parse the tag overview page to extract all links to articles of interest:
Second, we download and scrape each individual article page. For this, we extract all href
-attributes from a
-elements fitting a certain CSS-class. To select the right contents via XPATH-selectors, you need to investigate the HTML-structure of your specific page. Modern browsers such as Firefox and Chrome support you in that task by a function called “Inspect Element” (or similar), available through a right-click on the page element.
Now, links
contains a list of 20 hyperlinks to single articles tagged with Angela Merkel.
But stop! There is not only one page of links to tagged articles. If you have a look on the page in your browser, the tag overview page has several more than 60 sub pages, accessible via a paging navigator at the bottom. By clicking on the second page, we see a different URL-structure, which now contains a link to a specific paging number. We can use that format to create links to all sub pages by combining the base URL with the page numbers.
Now we can iterate over all URLs of tag overview pages, to collect more/all links to articles tagged with Angela Merkel. We iterate with a for-loop over all URLs and append results from each single URL to a vector of all links.
An effective way of programming is to encapsulate repeatedly used code in a specific function. This function then can be called with specific parameters, process something and return a result. We use this here, to encapsulate the downloading and parsing of a Guardian article given a specific URL. The code is the same as in our exercise 1 above, only that we combine the extracted texts and metadata in a data.frame and wrap the entire process in a function-Block.
Now we can use that function scrape_guardian_article
in any other part of our script. For instance, we can loop over each of our collected links. We use a running variable i, taking values from 1 to length(all_links)
to access the single links in all_links
and write some progress output.
The last command write the extracted articles to a CSV-file in the data directory for any later use.
Try to perform extraction of news articles from another web page, e.g. https://www.spiegel.de or https://www.nytimes.com.
For this, investigate the URL patterns of the page and look into the source code with the `inspect element’ functionality of your browser to find appropriate XPATH expressions.
2020, Andreas Niekler and Gregor Wiedemann. GPLv3. tm4ss.github.io
So you just discovered web scraping and you’re excited to get started on your first web scraping project.
But sometimes, it’s hard to get your creative juices going and come up with an idea for your first project.
Today, we will propose a couple of ideas that can get you started with web scraping.
What is Web Scraping?
Before we get started, you might be wondering what web scraping is in the first place. In short, web scraping refers to the extraction of data from a website on to a more useful format.
Web Scraping Exercises Pdf
In most cases, web scraping is done with an automated software tool rather than manually. If you’d like to learn more about web scraping, check our in-depth guide on web scraping and what it used for.
Web Scraping Ideas
We have put together 5 different ideas for you to start your first web scraping project.
We have built some of these examples to also allow you to realize the power of web scraping with further analysis.
Taking Price Comparison to the Next Level
One project a lot of people like to start with involves scraping ecommerce sites for product data and price comparison. While this project is a good place to get started, we suggest you take it to the next level and analyze the data from your scrape to find the best purchase in a certain category.
For example, you could scrape data about all tablets available on Amazon and analyze the dataset to figure out what is the best bang for your buck when comparing both pricing and review score data. You can make this analysis more detailed by filtering out products with a low amount of reviews.
You’d be looking to answer the question: What is the best rated tablet you can purchase for the lowest amount?
Ready to get started? Here’s our guide on how to scrape Amazon product data.
Build a Simple Investment App (No Coding)
This might project might sound a bit intimidating. However, building a simple investment app is easier than you’d think.
The goal of this app would be to setup your web scraper to scrape a few specific stocks from Yahoo Finance every day. This scrape will then be fed into a Google Spreadsheet and once any stock drops under a specific price, a “buy” notification will be sent to your email.
You can start this project by checking out the following quick guides:
Scrape a Subreddit to Find Popular Topics and Words
If you’re like me, you might have a few subreddits that you love to browse.
Do you sometimes wonder if there are specific words or topics that get more upvotes than others within that community? Or which topics get more comments and create more discussion?
You could scrape this subreddit and create graphs such as word-clouds to present the insights you’ve found.
You could then take these graphs and insights from your project and share them with that specific subreddit to spark further conversations (and get some sweet reddit karma!).
Interested in this project? Check out our guide on how to scrape reddit data.
Scrape a Leads Database for Someone Else (or sell it!)
You might know someone in your family or circle of friends who runs their own business. Why not help them by building a database of leads for their business?
First, you’d have to ask them about the details of their business and what kind of leads they would find valuable. After this, you can setup your web scraper to scrape leads data from the internet to build your database.
If you do not know anyone in your circle that might need a leads database, you could also try to sell it!
Want to complete this project? Here’s our guide on how to use a web scraping for lead generation.
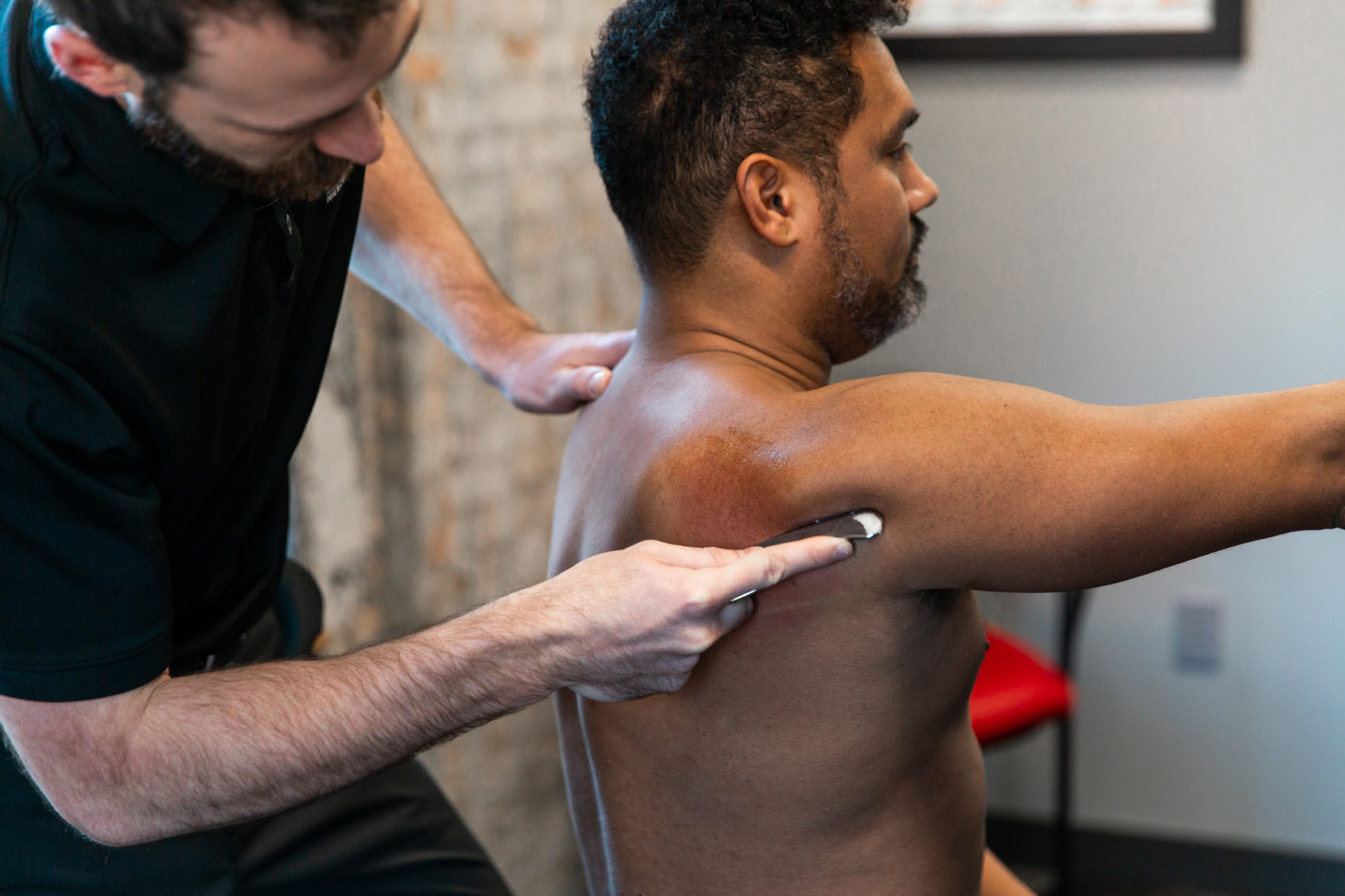
Web Scraping Software
Take on a Real Web Scraping Job
Web Scraping Exercises Pdf
Why not get started with a real-world example of a web scraping job?
Numerous one-off web scraping jobs get posted on job boards every day. These are great to get started with, since they are examples of what web scraping is being used for in the real world.
A great place to start is UpWork, where you can search for “web scraping” jobs and apply to take them up or just complete them regardless for learning purposes.
Web Scraping Service
Here’s the search results page for “web scraping” in UpWork.
What Web Scraper Should You Use?
At this point, you might already know what your first web scraping project will be.
Web Scraping Applications
However, you might still be wondering what web scraper you should be using to carry out your project. The truth is that the best web scraper for your project might be different depending on the needs of your project.
Web Scraping Software Comparison
However, every single project on this list can be completed using ParseHub, a powerful and free web scraper.
Web Scraping Exercises Free
What web scraping project will you tackle first?
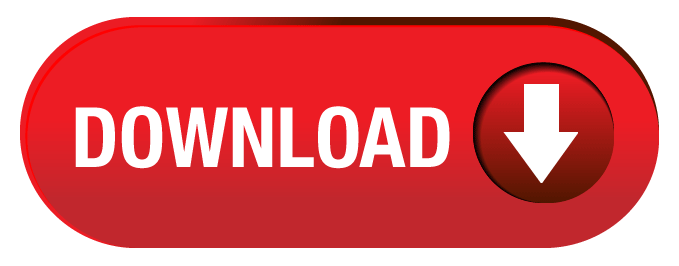